The AI Revolution
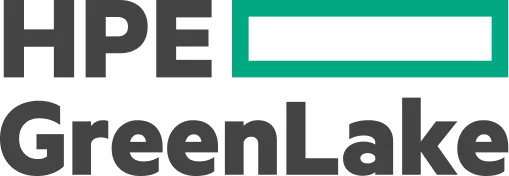
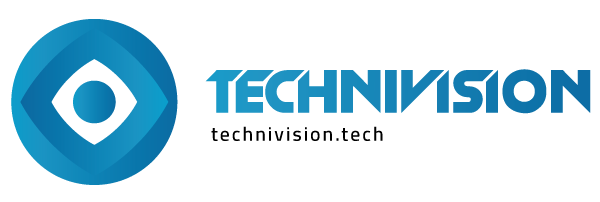

The AI Revolution
Embracing the AI Wave
In the midst of the current AI frenzy, it's crucial to recognize that artificial intelligence is not a novel concept. Originating in the 1950s, AI has gradually permeated various applications over the years. While many have experienced its impact through features like autocorrect, chatbots, or search engines, AI predominantly functioned in the background, providing assistance without significantly transforming user interactions. The landscape changed dramatically with the advent of DALL-E 2 and ChatGPT.
These innovations, which employ AI to generate images and content, democratized generative AI capabilities, making them accessible to individuals, including schoolchildren. As a result, AI has become ubiquitous in our daily lives.
Paving the Way for the Age of Insight
Antonio Neri, President and CEO of HPE, underscores the rapid acceleration of AI, attributing it to advancements in knowledge, software, and supercomputing capabilities. According to Neri, the true power of AI lies in its capacity to convert questions into discoveries, insights into actions, and unlimited imagination into reality.
He envisions AI ushering in an era characterized by profound insights and transformative capabilities.
Demystifying AI
Given the prominence of AI in corporate discussions, it is beneficial to grasp the essence of AI for effective engagement. At its core, AI emulates human intelligence to process data. NASA, for instance, leverages AI not only as a spacecraft pilot but also to streamline human resources transactions, leading to cost savings.
Unraveling the Machine Learning Lifecycle
Machine Learning (ML), a subset of AI, operates by developing models rather than explicit programming. Developers create an ML model for specific tasks, such as image recognition or spam detection, and train it with substantial datasets. The ensuing stages of the ML lifecycle involve careful data selection, algorithm choice, model construction, iterative training, and validation.
The ultimate goal is to deploy well-performing models in the real world, necessitating ongoing monitoring to ensure the algorithms function as intended.